Rayan Sadri (CEO) and Ali Rouzbayani (CTO) came to Canada from Iran several years ago to pursue studies at McGill University in Montreal. Ali is a biomedical engineer. Rayan is a software engineer. Together, they have been working on artificial intelligence (AI) applications to democratize healthcare with a mission to change the way life sciences work by building a privacy-first AI company after seeing how the lack of diverse, high-quality imaging datasets was slowing down AI advancements in the life sciences. This has led them to start Carez AI, pioneering clinically validated synthetic medical imaging to make data more accessible, scalable, and privacy-compliant for life science companies.Â
In this, his first contribution to the 21st Century Tech Blog, Rayan describes how, through their research, they hope to reshape the very fabric of medical diagnostics.Â
Imagine a world where doctors will deliver diagnoses on life-threatening diseases by practicing using thousands of hyper-realistic medical scans. They’ll be able to train using these images before ever seeing a patient. This isn’t science fiction. This is the promise of generative AI in medicine, not to replace reality, but to enhance it and ultimately save lives.
Carez AI is a system powered by generative AI that creates hyper-realistic MRI, X-ray, CT, ultrasound, and pathology data at scale. It allows users of its AI tool to turn rare, difficult-to-capture diseases into 20,000 perfectly annotated medical imaging samples with 98% accuracy. The best radiologists struggle to differentiate the medical imaging samples from real scans.
Why do this?
AI is increasingly seen as a gamechanger for medical diagnostics described as an industry-wide paradigm shift. When building these generative AI and machine learning tools, data is the key. The more test results and images scanned means greater identification and AI predictive capacity. That’s why thousands of teams are working in the medical field to develop AI diagnostic tools.
What’s slowing them down: data scarcity because clinical-grade AI only exists from datasets that are ethically sourced and representative of the demographics, anatomy, and medical conditions of an entire population.
Obtaining such datasets can cost a lot of money. Training per dataset can take up to a year. Even after all that, the data can still have critical gaps due to geography, population source and other systemic biases.
The way OpenAI and other AI developers have approached obtaining data for their AI tools to use for training often involves scraping the entire content of web pages without the permission of the content creators. This has led to lawsuits from the publishers of the content and concerns about invasion of privacy. When dealing with healthcare data, patient privacy is a big thing. In the U.S., HIPAA, in Canada, PIPEDA and in the European Union, GDPR rules govern the collection, digitization and sharing of patient data.
Carez AI has recognized that in developing its diagnostic tool that patient data privacy is paramount. Instead of a cookie-cutter model based on publicly scraped Internet data, it is working with users to create task-specific models based on their proprietary data, secured and insulated to safeguard patient privacy, copyright and model access. Every dataset generated is legally obtained with fully compliant data agreements.
The result is an AI diagnostic tool that fills the gaps found in proprietary datasets through the generation of anatomically accurate synthetic images. These synthetic images reflect population and geographic diversity with no systemic biases. When recently tested by five chief radiologists, the doctors could not distinguish Carez AI synthetic scans from real ones.
What does this mean? Simply put, it means a fully customizable, high-quality, synthetic dataset for diagnostic imaging that can be produced in minutes. To achieve these results, Carez AI has been working with the top companies in medical imaging. It was invited to present last year at the largest medical imaging conference in Europe, the Cardiovascular and Interventional Radiological Society of Europe (CIRSE 2024), where it was chosen as one of the top AI startups in the field.
What is the future of synthetic imaging diagnostic AI tools? Advancements in generative AI and machine learning are seen by the healthcare sector as an increasingly attractive diagnostic imaging option. Market predictions (see graph below) project annual growth of 35.3% through 2030 for this burgeoning field.
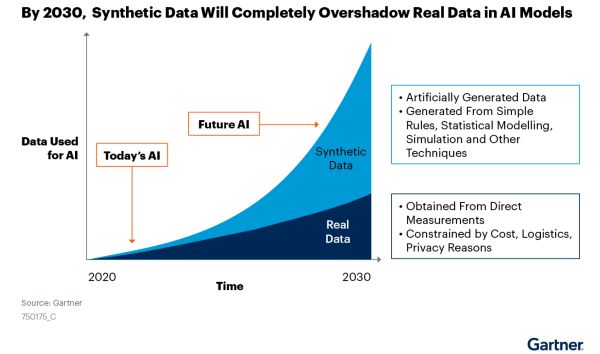
Recently, Gretel, a company acquired by NVIDIA, has been accelerating the trend in the adoption of synthetic data. Gretel supports various data types, including tabular, unstructured, time-series, relational, and image data. Carez AI remains focused on diagnostic imaging data tools to save lives. It sees synthetic imaging datasets as a key to democratizing healthcare delivery, leveling the playing field for hospitals worldwide, whether located in Montreal, San Francisco, or rural Uganda.